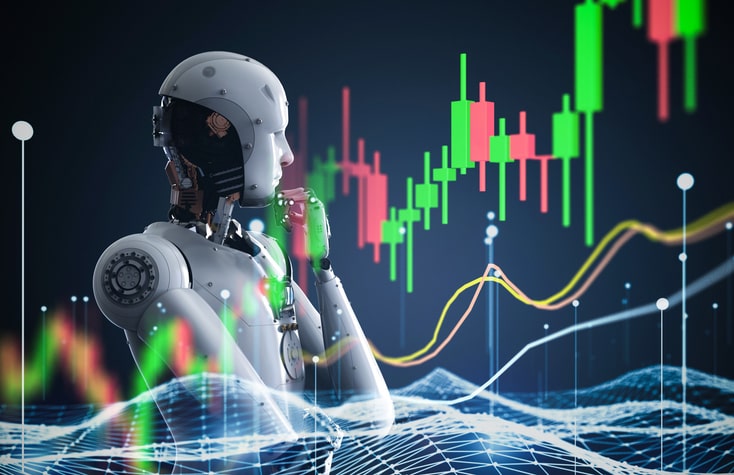
An open-source tool is a software program whose source code is made available to the public for free. This means anyone can view, modify, and distribute the code as they see fit. Open-source tools are typically developed collaboratively by communities of developers, allowing for continuous improvements and innovations. Artificial Intelligence (AI) can be categorized into various types based on capabilities and functionalities.
Based on Capabilities, we have:
- Narrow AI (Weak AI):
- Definition: AI that is designed to perform a specific task or a narrow range of tasks.
- Examples: Voice assistants (e.g., Siri, Alexa), recommendation systems (e.g., Netflix, Amazon), and facial recognition systems.
- General AI (Strong AI):
- Definition: AI that possesses the ability to understand, learn, and apply knowledge across a wide range of tasks, similar to human intelligence.
- Examples: Currently hypothetical and not yet realized. It would be an AI that can perform any intellectual task that a human can do.
- Super AI:
- Definition: AI that surpasses human intelligence and capabilities in all aspects.
- Examples: Purely theoretical at this point. It would be an AI that outperforms the best human minds in every field.
Based on Functionalities, we have:
- Reactive Machines:
- Definition: AI systems that can only react to specific inputs and do not have the ability to learn from past experiences.
- Examples: IBM’s Deep Blue, the chess-playing computer.
- Limited Memory:
- Definition: AI systems that can learn from historical data to make decisions and improve over time.
- Examples: Self-driving cars, which use data from past trips to navigate current routes.
- Theory of Mind:
- Definition: AI systems that can understand human emotions, beliefs, and social interactions.
- Examples: In developmental stages, with research ongoing to achieve this level of understanding.
- Self-Aware AI:
- Definition: AI that possesses self-awareness and consciousness, similar to humans.
- Examples: Currently theoretical and not achieved.
AI and open-source tools are revolutionizing forensic accounting and valuation services in several ways:
AI in Forensic Accounting
- Data analysis and pattern recognition: AI and machine learning (ML) algorithms can quickly process and analyze large volumes of financial data, identifying patterns and anomalies that might indicate fraudulent activities and go unnoticed in a manual review.
- Natural language processing: Commonly referred to as GenAI. AI and machine learning (ML) algorithms can assist in reviewing voluminous documents, emails, chats, and transcripts as part of an investigation. This is the area that is most commonly used for forensic accounting.
- Risk assessment identification: AI can automate the categorization of financial transactions based on risk levels, allowing forensic accountants to focus on high-risk areas that require closer scrutiny.
- Real-Time Monitoring: AI systems can monitor transactions in real-time, immediately flagging suspicious activities and enabling faster responses to potential fraud.
Open-Source Tools in Valuation Services
- Automated Valuation Models (AVMs): Open-source AVMs utilize advanced ML techniques to estimate property values accurately. These models can process large datasets and provide reliable valuations for real estate properties.
- Cost Efficiency: Open-source software is often more cost-effective than proprietary solutions, making it accessible for smaller firms and individual practitioners.
- Transparency and Collaboration: Open-source tools allow for greater transparency and collaboration among developers and users, leading to continuous improvements and innovations.
Benefits and Risks
- Benefits: AI and open-source tools enhance the speed, accuracy, and efficiency of forensic accounting and valuation services. They enable professionals to handle large datasets, detect fraud more effectively, and provide accurate valuations.
- Risks: The misuse of AI for fraudulent purposes and the potential for errors in open-source software are concerns that need to be addressed. Proper training, oversight, and continuous updates are essential to mitigate these risks. AI is not the be-all and end-all, and we are still responsible for the input. Understanding the source that is feeding the AI is critical.
Concerns with using AI and Open-source tools in Forensic Accounting and Valuation Services
- Confidentiality and privacy: The best practice is not to widely adopt AI or open-source tools without ensuring that the applications used are completely secure. For example, using AI in a litigation setting or legal filing can raise concerns when there is any personal identifying information (PII).
- Ethical considerations: There is always a risk related to plagiarism. It’s important to cite your sources when you use AI for deep-dive analytics for forensic, research, or business valuation. Tools like Gemini or Claude.ai are pretty good at scouring the web to find the sources.
- Basic understanding of your expected output: Understanding your expectations is key to evaluating the results produced by AI queries. It’s not best practice to rely on the AI results without having your knowledge of what could be the answer. Using AI and open-source tools must be in addition to your basic understanding of the subject matter, not a “plug and play” alternative.
- Overreliance on AI: It’s important to make a difference between “using AI” and “relying on AI.” Economic & industry research and the automation of routine tasks could be effective ways to use AI tools in Forensic Accounting and Valuation Services. Creating a process or policy for using AI will put you in a position of overreliance on AI, which could pose a potential risk that we discussed above.